AI Marketing Tools To Help You Win in 2024
Top AI marketing tools by category
Forgive us for using a technical term, but it’s absolutely bananas how far AI has come in the past year. And while some people are worried that the robots are here to take our jobs, we’re convinced they’re here to help us do our jobs better—especially when it comes to AI marketing tools.
If you are a small team or solopreneur wanting to scale your reach and analytics capability, AI marketing tools can be a game-changer. With the right software, companies with minimal resources can compete with the big boys.
AI marketing software can help draft content, generate SEO-friendly social captions, repurpose a blog post into a video, suggest an ad strategy, and so much more. Seriously, just imagine what your marketing team could accomplish with a little (digital) helping hand.
Ready to step up your social media marketing with a little help from artificial intelligence? Read on for our master list of the best AI marketing tools for 2024.
Bonus: Download this free cheat sheet with 250 professionally engineered ChatGPT prompts that will help you be more productive at work and in your daily life. We’ve included specific prompts for over 25 industries and professions!
25 best AI marketing tools for 2024
A quick caveat before we dive into our list of the best AI marketing tools for 2024: the “best” software for you is going to be totally dependent on your social media goals.
So before you lean into the world of automation and adopt every tool here, take a minute to think about what parts of your current process could use scaling or streamlining.
1. Hootsuite
Managing social media = 25 jobs. Creating content, posting to multiple networks, learning about your audience, providing customer support, reporting wins to your boss… It’s a lot.
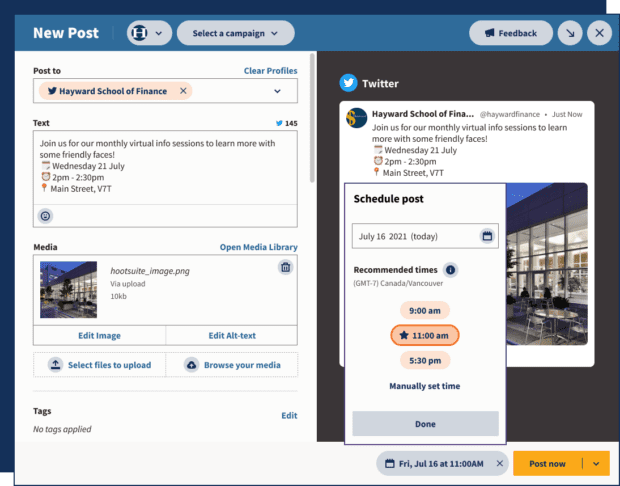
Hootsuite can help you do all of that (and more) from one user-friendly dashboard that works with Facebook, Instagram, X (formerly Twitter), LinkedIn, TikTok, Pinterest, and YouTube.
With Hootsuite, you get:
- An easy-to-use social media scheduler
- Personalized recommendations for best times to post
- Intuitive analytics for all your social accounts
- One inbox for DMs and comments from every network
- Industry benchmarks and competitor analytics
- Simple social media monitoring tools
- Integrations with all of your other tools, including Canva, Hubspot, Shopify, Mailchimp, Microsoft Dynamics, and 200+ more
But hey, this is a story about AI marketing tools… and Hootsuite absolutely delivers there, too.
OwlyWriter is built on ChatGPT’s pioneering language model, but it also includes all of our winning content formulas that took over 14 years of research to develop.
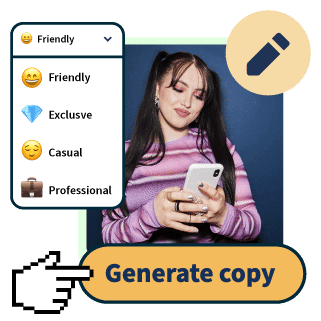
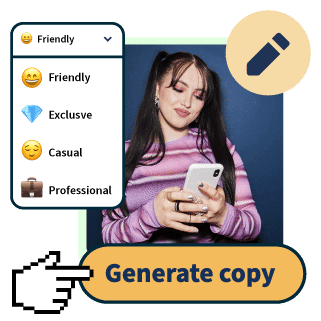
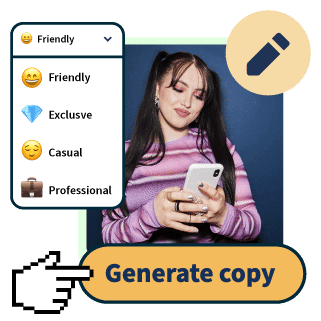
Craft perfect posts in seconds
OwlyWriter AI instantly generates captions and content ideas for every social media network. It’s seriously easy.
OwlyWriter can:
- Write a new social media caption in a specific tone of voice
- Write a post based on a link (e.g., a blog post or a product page)
- Generate post ideas based on a keyword or topic (and then write posts expanding on the idea you like best)
- Identify and repurpose your top-performing posts
- Create relevant captions for upcoming holidays
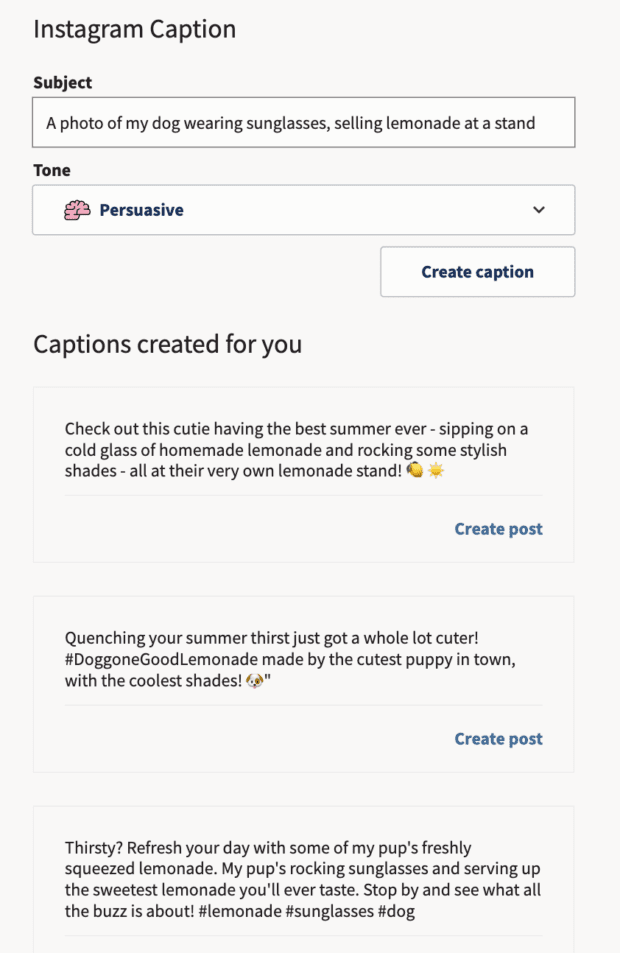
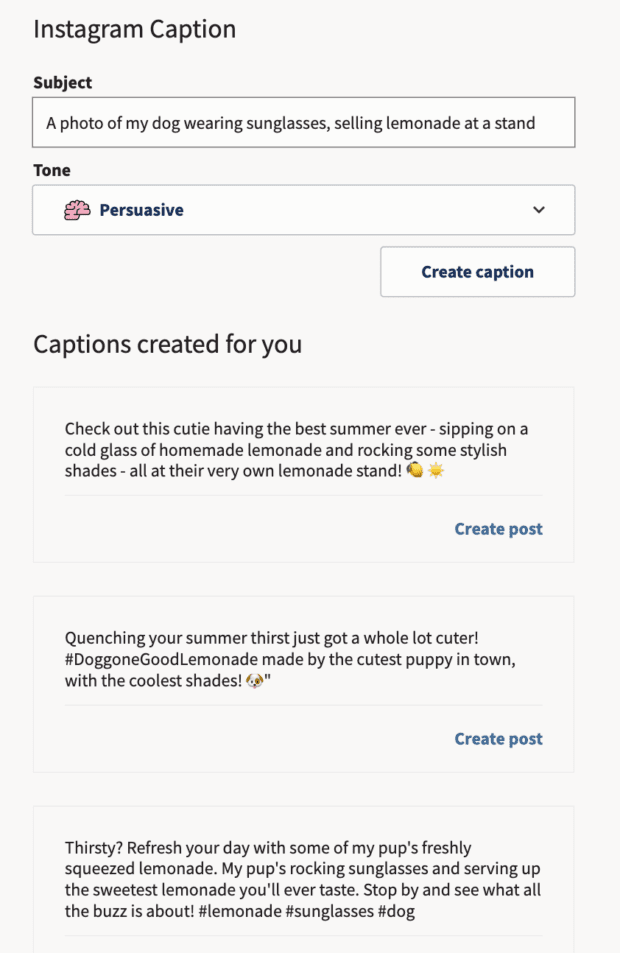
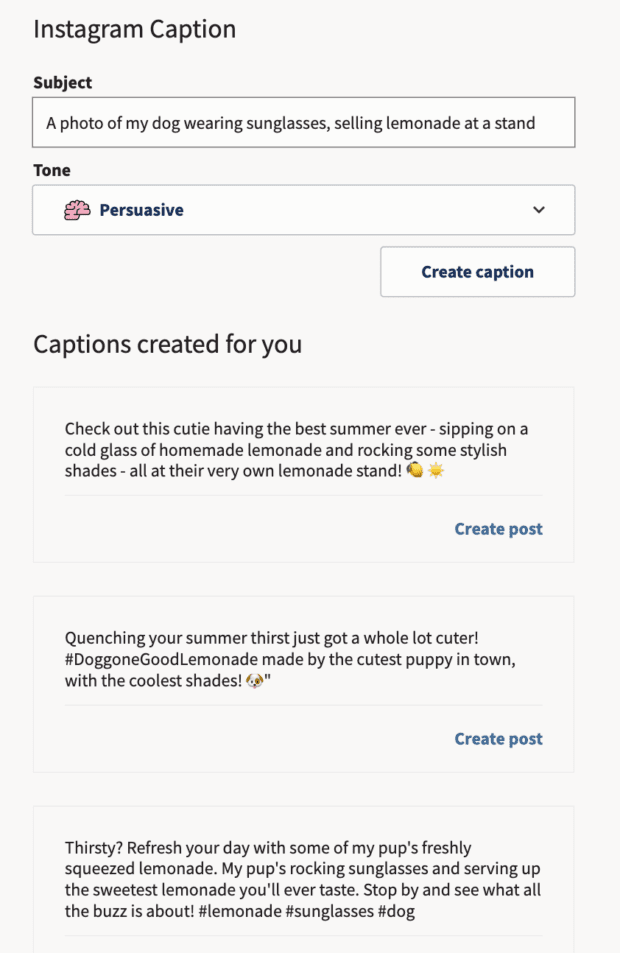
If you’re fine with writing your own captions but need a little help generating relevant hashtags, Hootsuite’s AI hashtag suggestion tool is a game-changer.
We all know hashtags are kind of a secret weapon when it comes to expanding your reach, but coming up with the right hashtags on your own can be…tricky.
Hootsuite’s AI suggestion tool is built right into the Compose window, so it’s super easy to generate smart hashtags while you write and schedule your content. Our AI technology analyzes both your caption and the images you’ve uploaded to suggest the most relevant hashtags.
And if you’re handling customer service via social media, Hootsuite Inbox also features some powerful AI capabilities. With Hootsuite Inbox, you can bridge the gap between social media engagement and customer service — and manage all of your social media messages in one place.
Beyond collecting all your DMs into a handy package, Inbox also features:
- Automated message routing
- Auto-responses and saved replies
- Automatically triggered customer satisfaction surveys
- AI-powered chatbot features
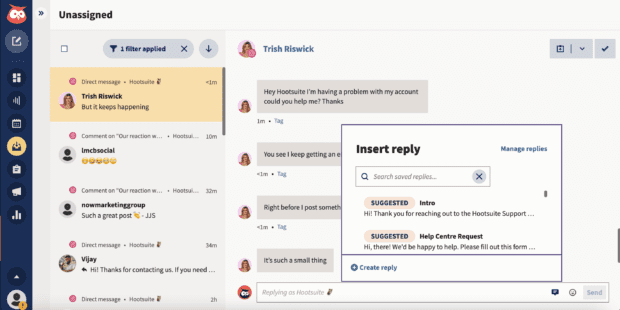
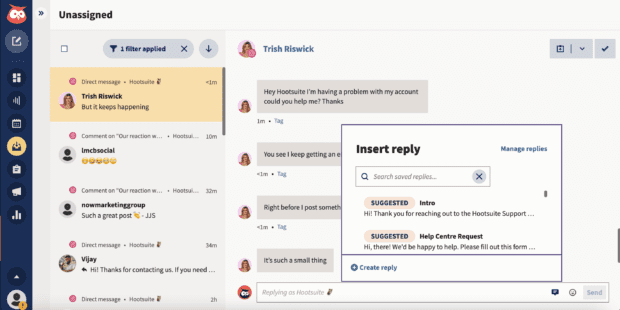
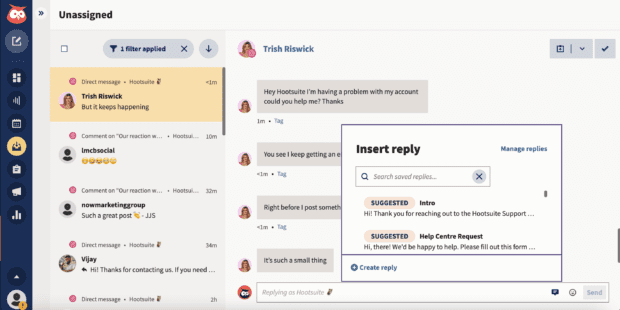
Take Hootsuite Inbox for a test drive
Price: Starts at $99 for professional plans
Who it’s best for: Social media managers, digital marketing professionals, small to large businesses, content creators
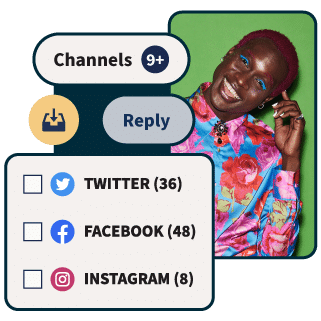
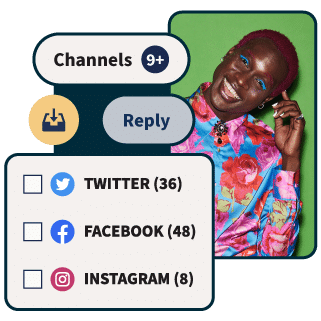
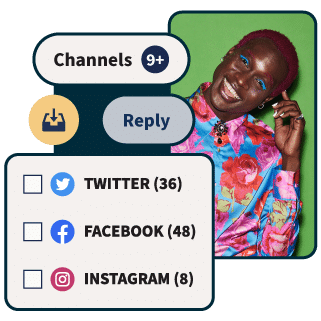
Reduce response time (and your workload)
Manage all your messages stress-free with easy routing, saved replies, and friendly chatbots. Try Hootsuite’s Inbox today.
2. Hootsuite’s free AI tools
Subscription plans gettin’ you down? Not to fear, Hootsuite has free AI content creation tools you can use right now:
- Caption generator. Curious about OwlyWriter AI or other AI writing tools? Try out caption generation for free. This generator isn’t as advanced as OwlyWriter. Still, unlike ChatGPT, it’s optimized for social media and custom-creates your caption for your chosen platform in one of five languages. Nice.
- Username idea generator. Identity crisis? No problem! With Hootsuite’s username generator, you can reinvent yourself in a few seconds flat.
- About/bio writer. We get it—it can feel awkward to write about yourself. Let Hootsuite sum up the special sauce of your life automatically.
- AI hashtag generator. Not sure how to tag your latest Insta post for maximum exposure? Our hashtag tool is here to help. (And if you want suggestions based on an image or video? Sign up for Hootsuite for the full multimedia experience!)
Price: Free!
Who it’s best for: Content creators, small teams, businesses just beginning their social media journeys
AI tools for content marketing
3. ChatGPT
Does ChatGPT even need an introduction? It’s become the default name for “AI content creation.”
A natural language processing (NLP) chatbot, ChatGPT can comprehend and generate material that sounds like a real person wrote it, including blog posts, social media postings and more.
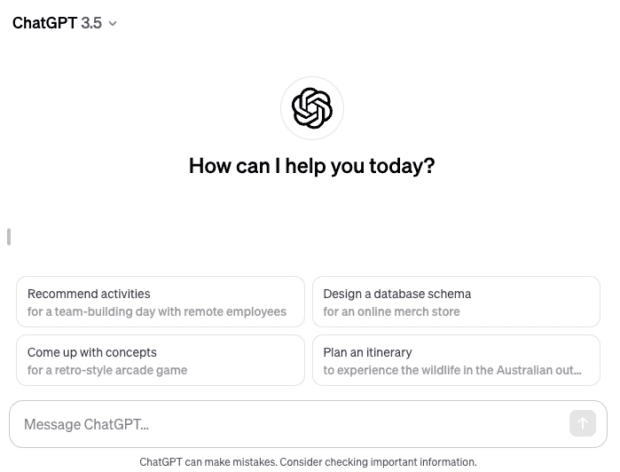
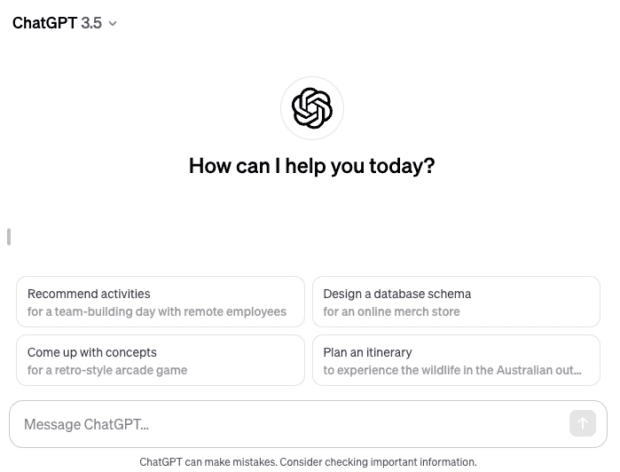
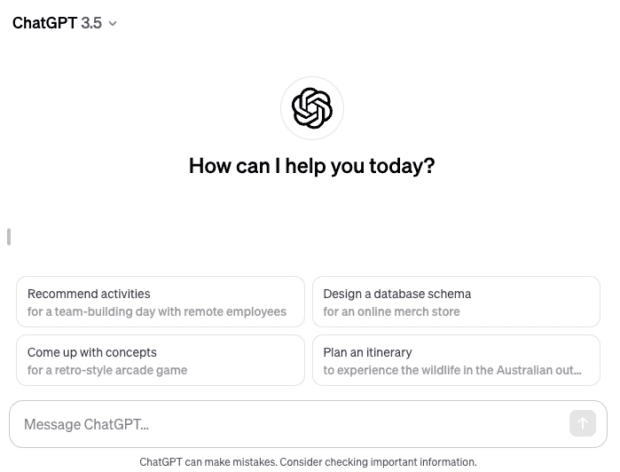
Price: Free! The “ChatGPT+” subscription costs $20 a month and offers faster response times and early access to new features
Who it’s best for: Developers, businesses with AI integration needs, content creators, customer support teams
4. Dall-E by Open.ai
Dall-E is the visual sibling of ChatGPT, an AI-powered solution for graphic design. Provide a text prompt detailing your visual concept, and Dall-E brings it to life.
Dall-E is great for crafting original graphics for blog posts or social media, prototyping designs, or developing web graphics.
It’s not the best for creating brand elements like logos or packaging designs, though. Brand identity, after all, calls for more than just aesthetics.
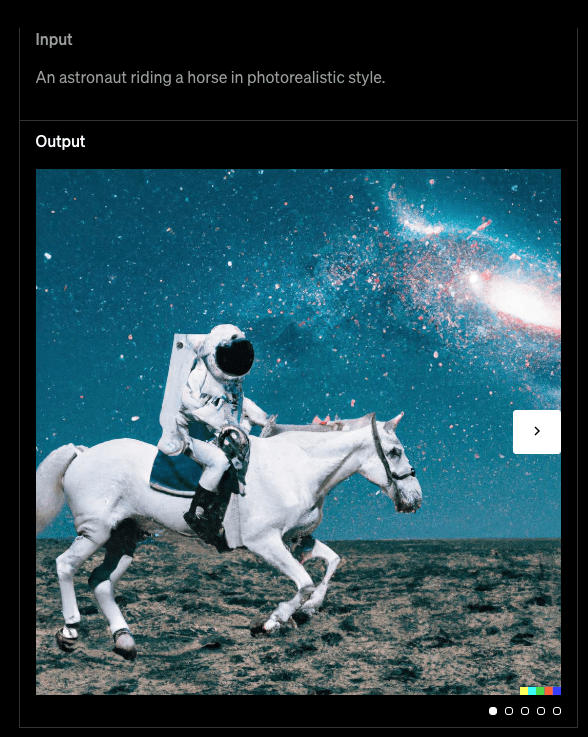
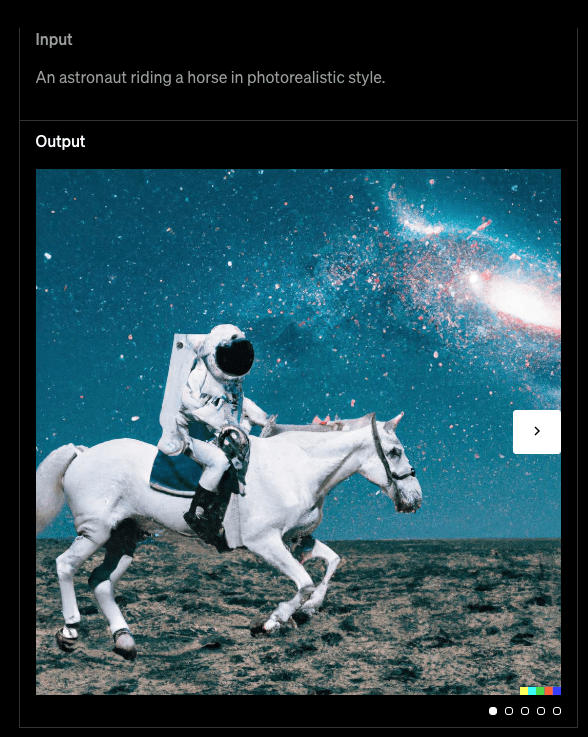
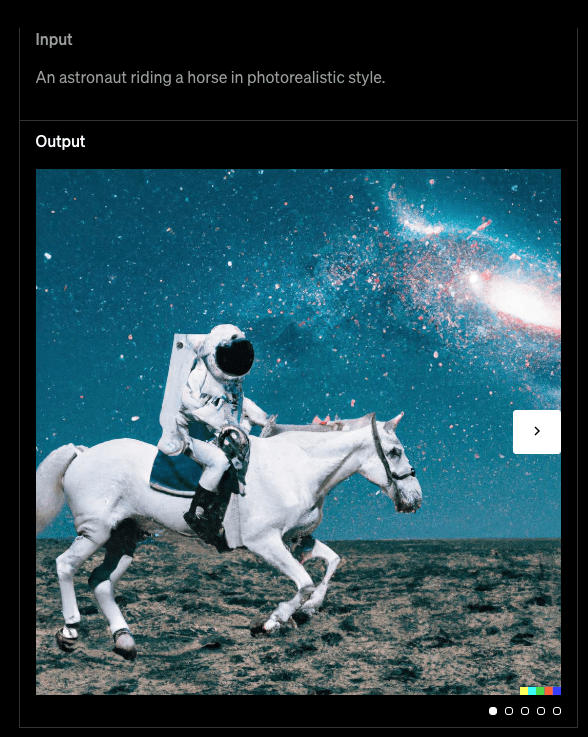
Price: Dall-e charges by the image, with the pricing ranging between $0.016 an image to $0.040 depending on the scale and resolution.
Who it’s best for: Graphic designers, artists, creatives, marketing professionals
5. Copy.ai
Copy.ai streamlines the copywriting process by generating almost-ready-to-publish drafts requiring minimal human editing.
Just provide a topic and creative direction; you’ll get outlines, articles, social posts, and sales emails in a few seconds. Try this AI marketing tool out if you need to generate written content rapidly at scale.
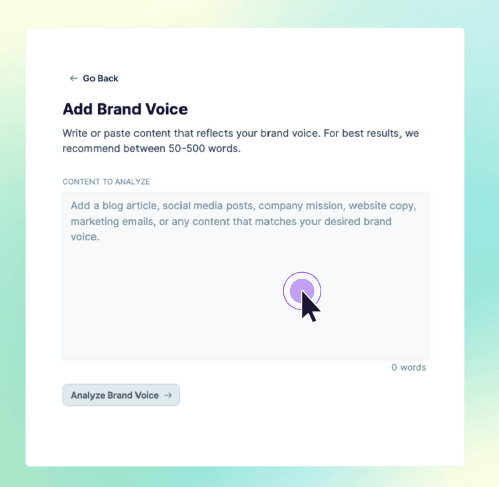
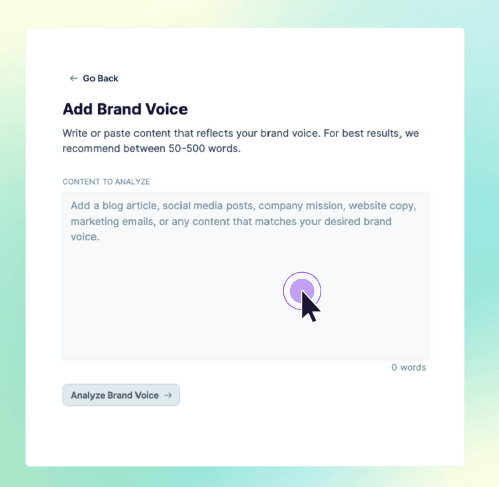
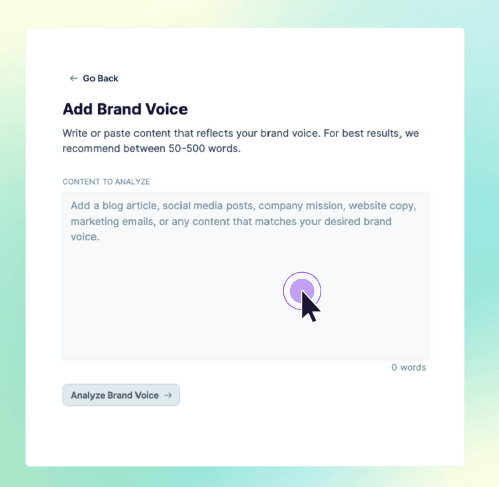
Price: The basic plan is free, with pro plans starting at $36 a month
Who it’s best for: Content writers, copywriters, marketers, small business owners
6. JasperAI
A major ChatGPT competitor, JasperAI’s specialty is its ability to account for tone. That makes it a great tool for generating on-brand articles, social media posts, and scripts that consistently reflect your voice.
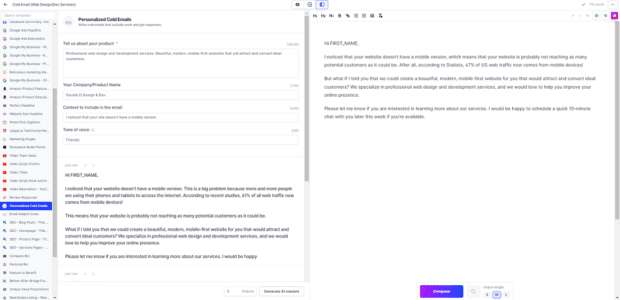
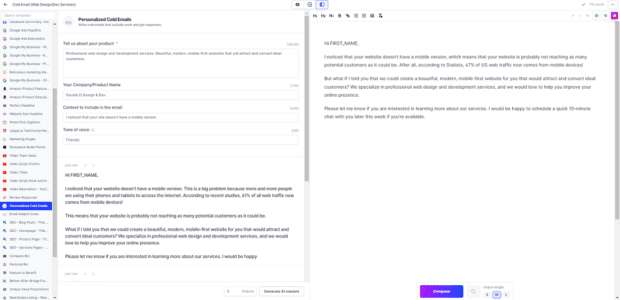
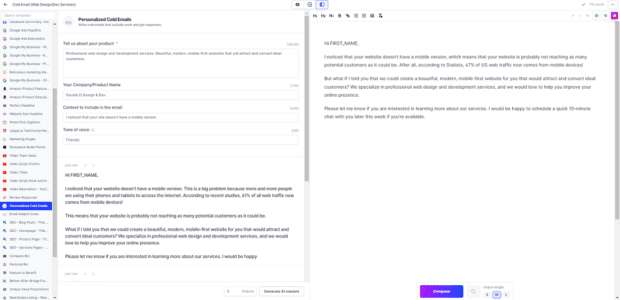
JasperAI also facilitates content translation into 30 languages and integrates with tons of different applications.
Try using JasperAI to generate content ideas and outlines or translate content for global audiences. (That being said, leave the big stories to human professionals.)
Price: From $39 a month
Who it’s best for: Individual content creators, collaborative marketing teams
7. Canva
Creating a brand from scratch? Work with a graphic design professional. Looking to repurpose existing content and maintain visual consistency using a library of graphic templates? Canva is a great tool to keep in your toolbox.
Canva isn’t just for social graphics; it covers document design, presentations, and more.
Plus, with the introduction of Magic Design, Canva utilizes AI-powered content creation to generate matching templates based on uploaded media, making it efficient to repurpose content across various platforms.
Price: A basic account is free, but to access premium content and new tools (like Magic Design), prices start at $18.99 a month
Who it’s best for: Designers, small business owners, social media managers, students
8. Midjourney
Similar to Dall-E, Midjourney creates graphics using AI prompts. It operates as a Discord bot, which means it’s very user-friendly, and you can enable art creation from anywhere—even your phone.
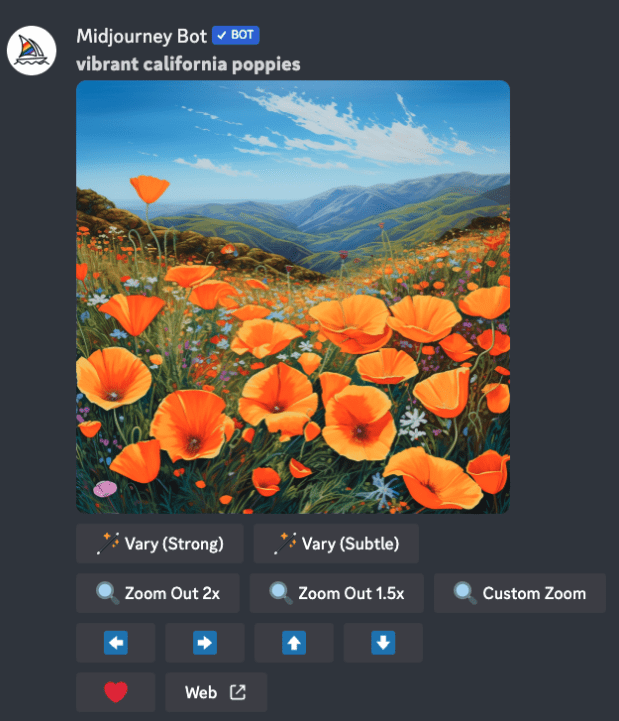
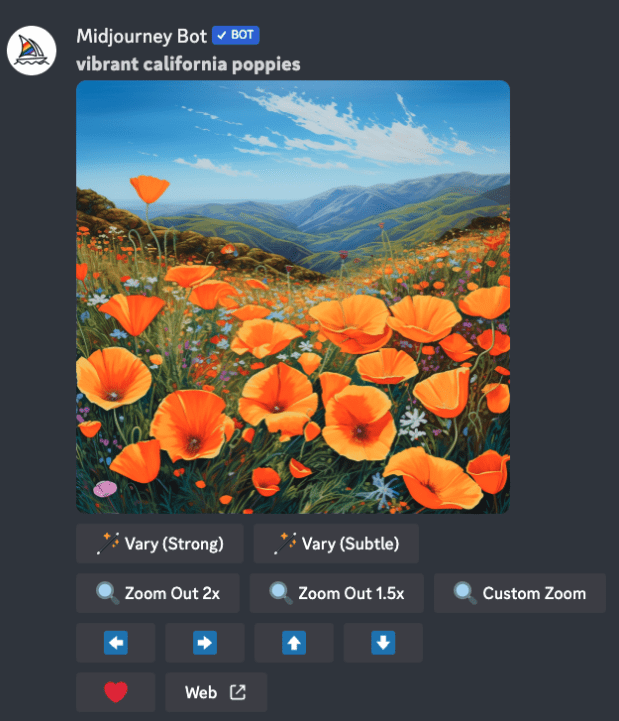
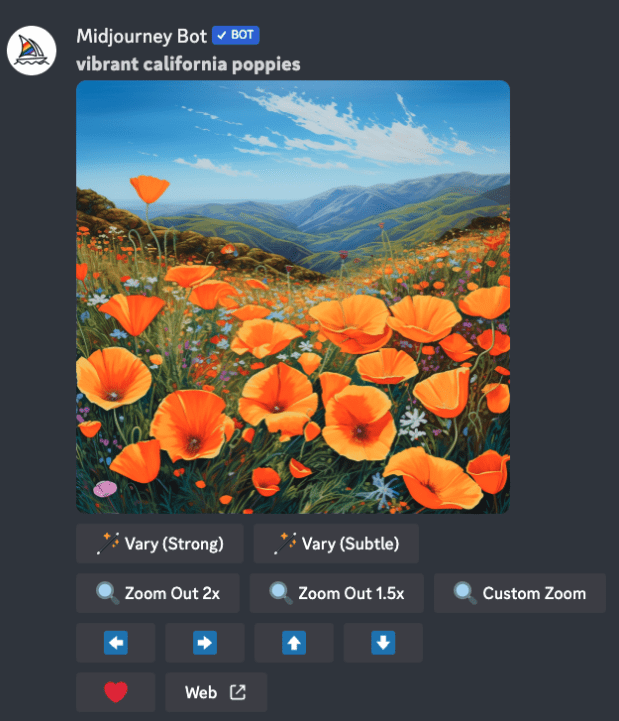
While anyone can learn to create prompts in Midjourney, getting the results you want takes a bit of practice—check out our AI art prompting guide.
Advanced Midjourney options include specifying features like a transparent background, aspect ratio, and art style.
Price: From $10 a month
Who it’s best for: Advanced AI image users, graphic designers
9. Synthesia
Synthesia enhances video production, even if you’re the most camera-shy marketing person on the planet. It uses AI avatars to quickly transform video scripts into finished videos.
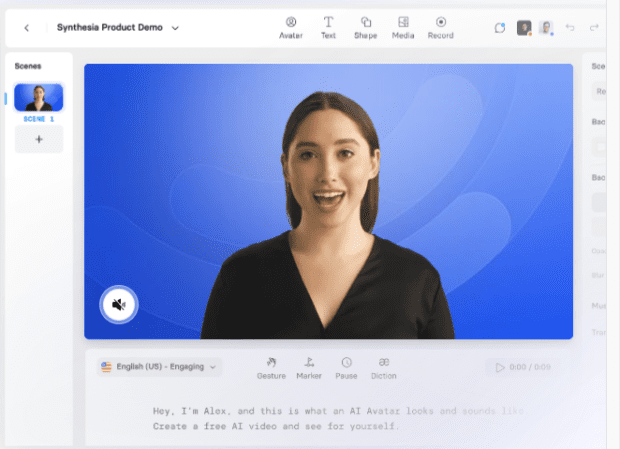
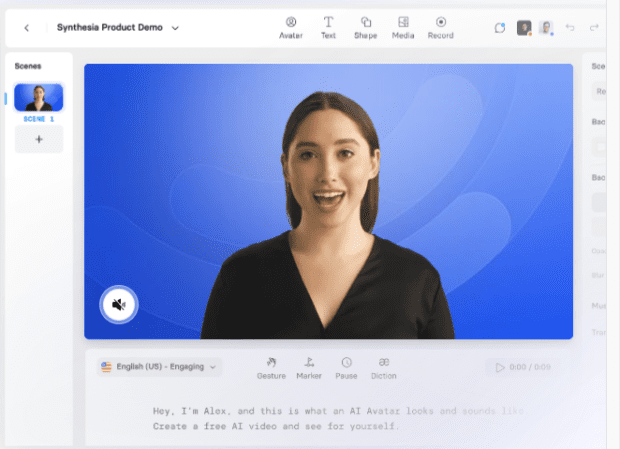
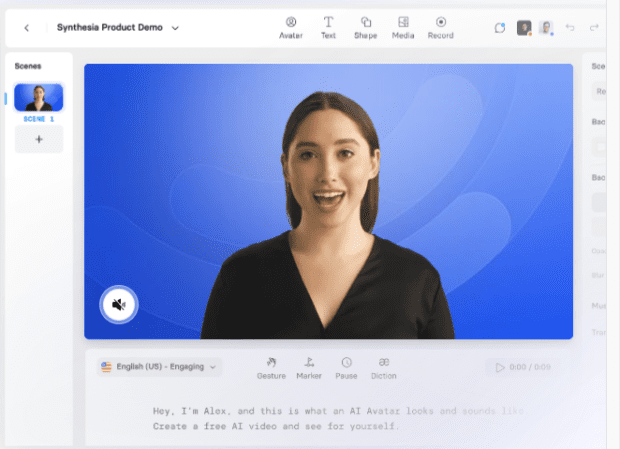
The platform offers industry-specific templates that facilitate quick script creation. Plus, users can review and edit the video before publishing, all within the software.
Use it to create quick how-to tutorials at scale or generate clips for Reels and TikTok.
Price: Starting from $22 a month
Who it’s best for: Video editors, marketing professionals
10. Murf
Murf specializes in generating real, human-sounding voices for reading scripts. The platform’s AI voices are created from real people, allowing you to produce studio-quality audio in 20 languages.
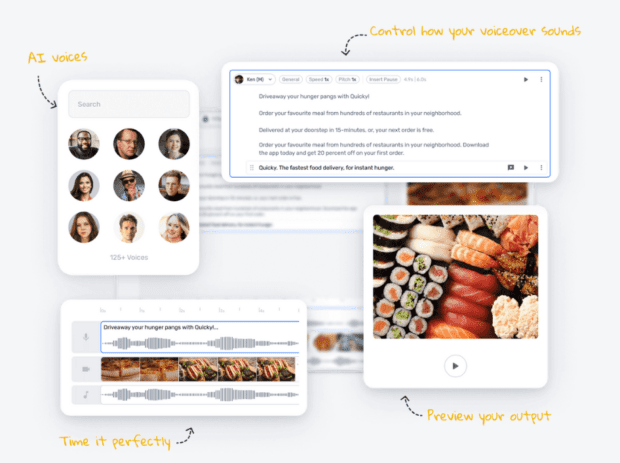
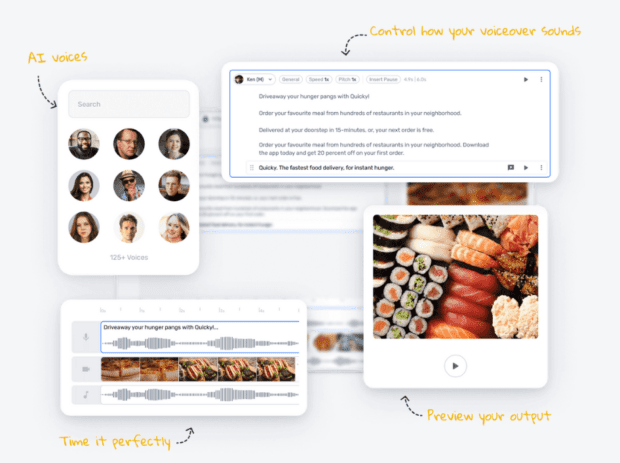
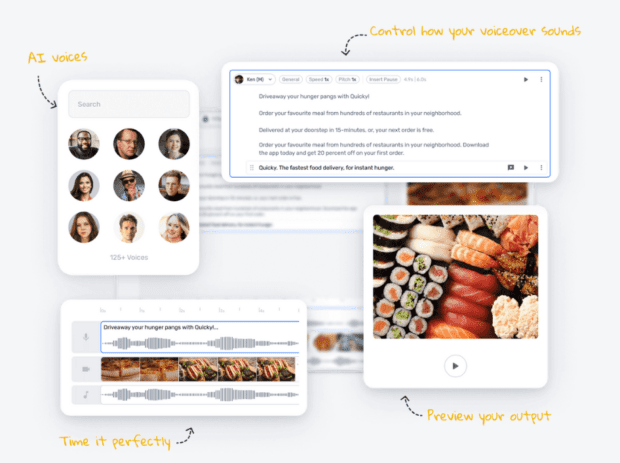
You can even clone your own voice for authenticity and time savings. It’s a great tool for recording voiceovers for social videos, podcasts, or brand presentations (though, as with many AI tools, it’s not the best for long-term branding).
Price: The entry level plan is free, with more advanced tools available starting from $19 a month
Who it’s best for: Teams interested in multimedia production
11. Podcastle
Looking into video or audio podcasts? Podcastle might be for you. This AI-powered podcast recording and editing app allows you to record video and audio streams, including virtual interviews with up to 10 participants.
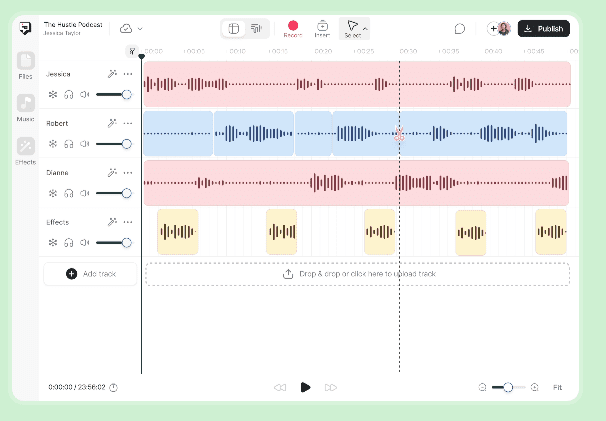
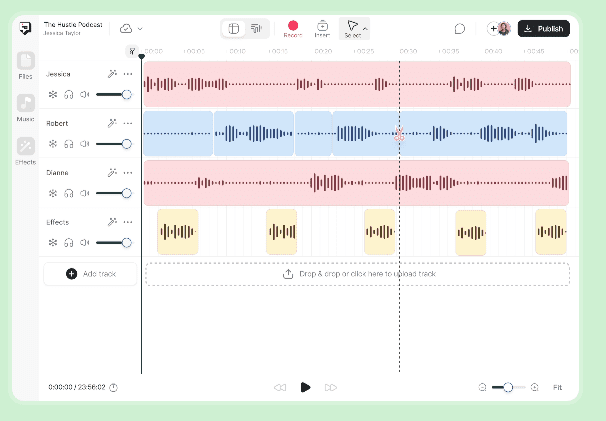
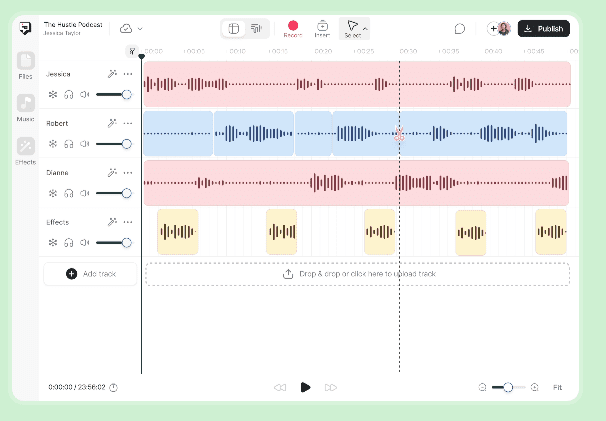
Podcastle’s AI-powered editing automates tasks like cutting out silence, suggesting clip trims, and minimizing noise while maintaining consistent volume levels.
In other words, this is a great option if you’re starting a new podcast and need easy audio and video editing tools (and a fancy voice-cloning feature). If you’re more experienced in the podcast world, though, this might be too basic for your needs.
Price: Basic plans are free, with more advanced functionality available from $12.99 a month
Who it’s best for: Beginning podcasters
12. Quillbot
QuillBot differentiates itself by helping you rephrase existing content creatively. It goes beyond basic synonym changes—QuillBot can simplify or expand content for brevity or detail.
It also offers unique extensions, including a web research AI search tool, a citation generator, and a “co-writing” sentence completer.
Use Quillbot to repurpose content or generate multiple versions of the same story.
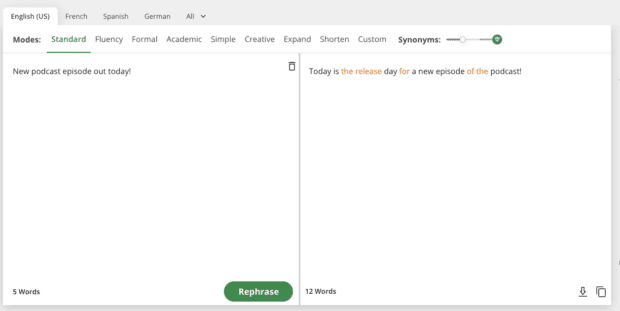
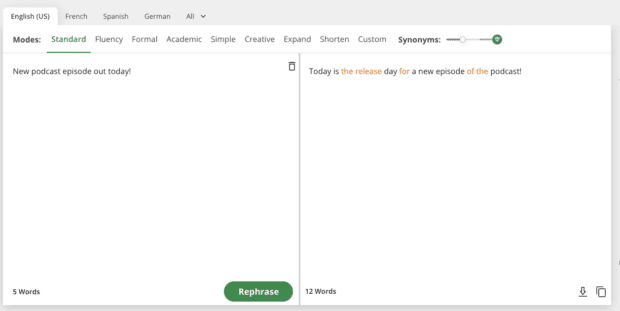
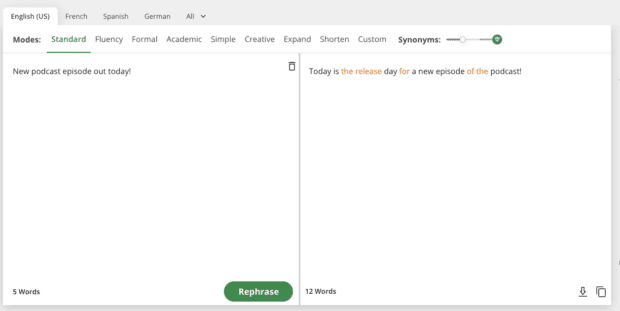
Price: Some functions available for free, but to access all the tools, plans start at $8.33 a month
Who it’s best for: Social media managers looking to repurpose existing content
13. Magic Studio
Wanna level up your product pics? Magic Studio is your new go-to. This AI tool caters to brands aiming to elevate their visual presence.
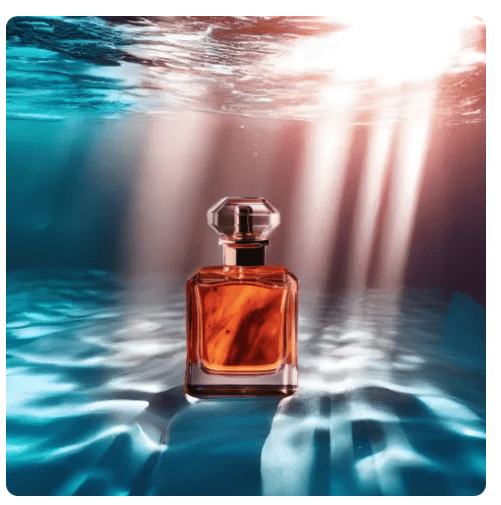
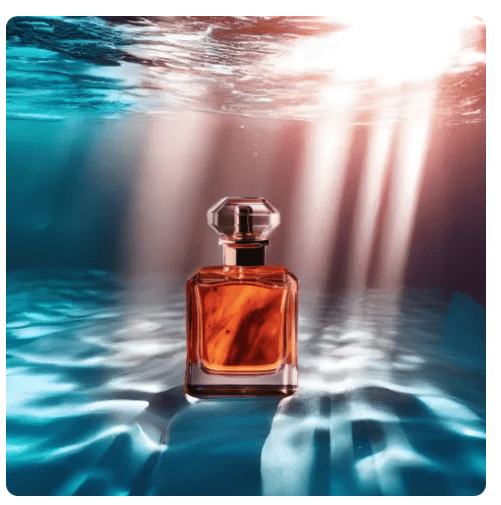
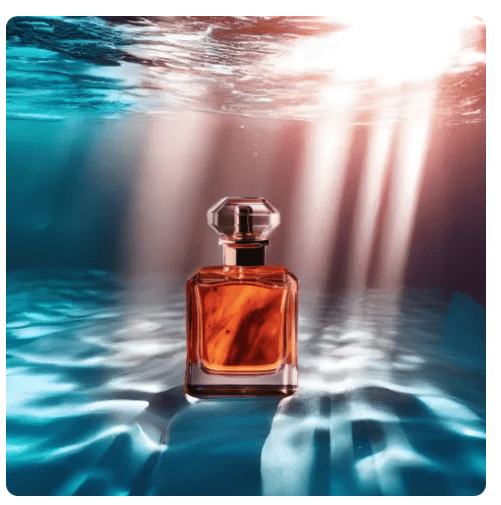
Magic Studio helps instantly place your best-sellers on sleek backgrounds, remove unwanted objects, and generate photos based on text prompts. It’s also a great tool for creating all-star profile pics.
Price: Some functions are free, but pro plans start from $7.49 a month
Who it’s best for: Ecommerce brands
14. DeepBrain AI Studios
DeepBrain AI Studios offers a user-friendly platform for AI video creation, converting text to video seamlessly. (It’s honestly a little spooky.)
Thanks to customizable photo-realistic AI avatars, the intuitive tool empowers beginners to create high-quality videos without actors, filming teams, or expensive equipment.
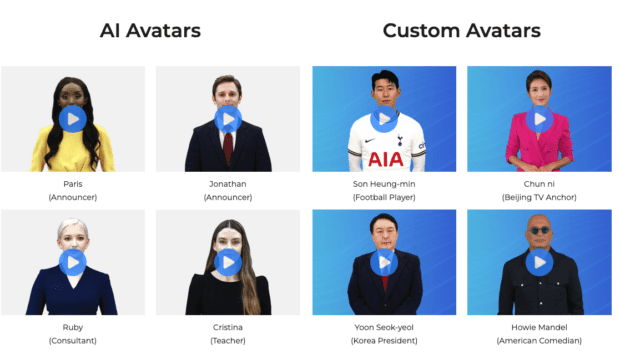
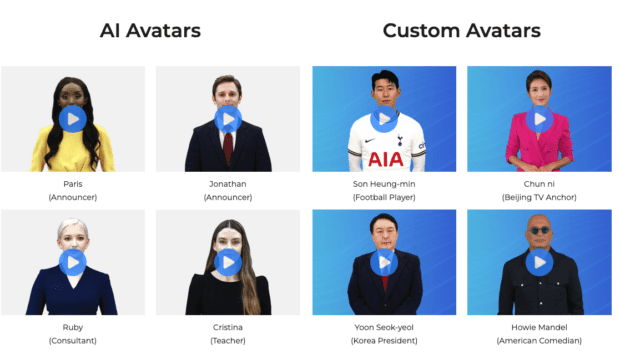
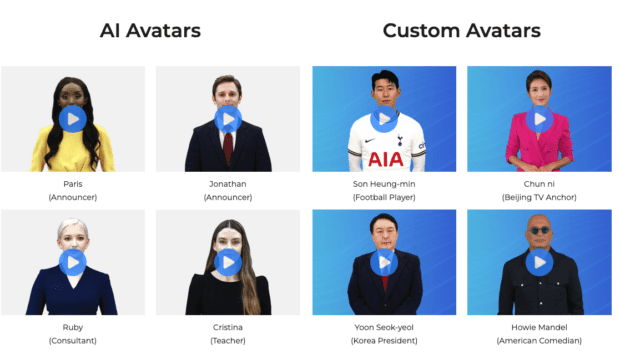
Price: From $29 a month
Who it’s best for: Video editors, brands with YouTube channels
15. Acrolinx
Acrolinx goes beyond just churning out content for blog posts. It serves as a brand watchdog, ensuring content aligns with brand guidelines. Set your style, tone, grammar, and specific language, and Acrolinx will help you generate content that never deviates from the brand vision.
Its AI Video Generator converts text to video, and photo-realistic AI avatars can be tailored to suit your brand.
Price: Price available upon request
Who it’s best for: Enterprise brands, marketing professionals
AI tools for SEO
16. Keyword Insights
Keyword Insights is a robust SEO tool that features an advanced AI writing assistant specifically tailored for contemporary content creators.
The integrated platform seamlessly combines content research, writing and search optimization. The writing assistant within Keyword Insights offers AI functionality while fostering a touch of human-AI collaboration.
Price: Access to some SEO tools are free, with a basic plan starting at $58 a month
Who it’s best for: SEO professionals, content marketers
17. Surfer SEO
Surfer SEO is a tool designed to enhance the content quality of web pages, ensuring higher rankings on search engine results pages (SERPs).
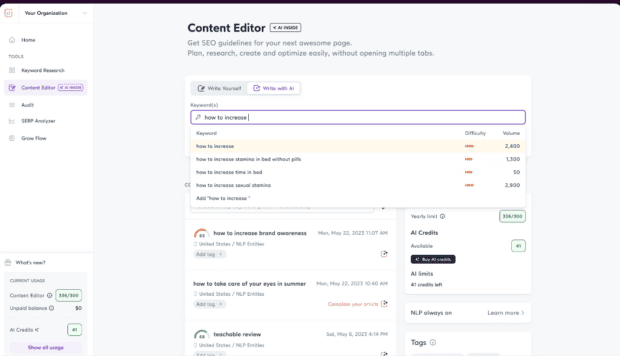
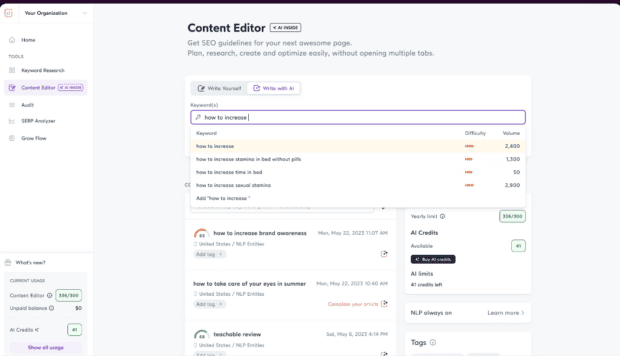
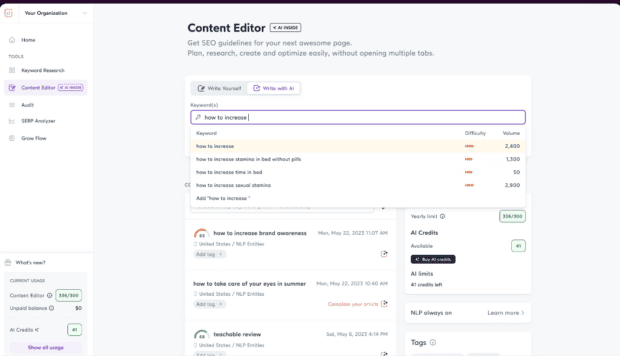
It meticulously analyzes SERPs for relevant search terms and compares your content against the insights gleaned from top-ranking pages. Surfer SEO evaluates keywords and various ranking metrics, providing you with valuable suggestions to optimize your content for search engine optimization. It also offers an outline generator and keyword research tools to help you create SEO-friendly content from the outset.
Price: Starting from $96.39 a month
Who it’s best for: Agencies, brands ready to scale
18. GrowthBar
GrowthBar employs GPT-3 AI technology to automate content generation and offers suggestions for keywords, precise word count, links, images, and more.
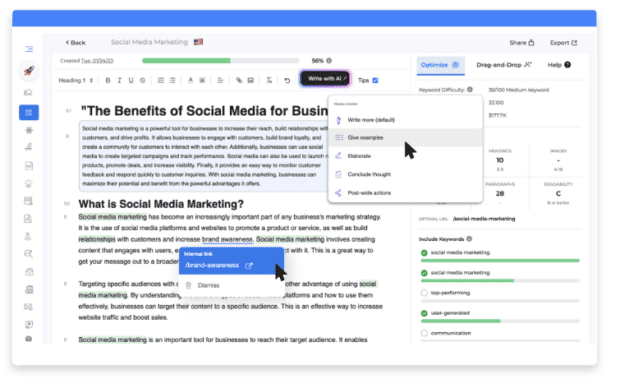
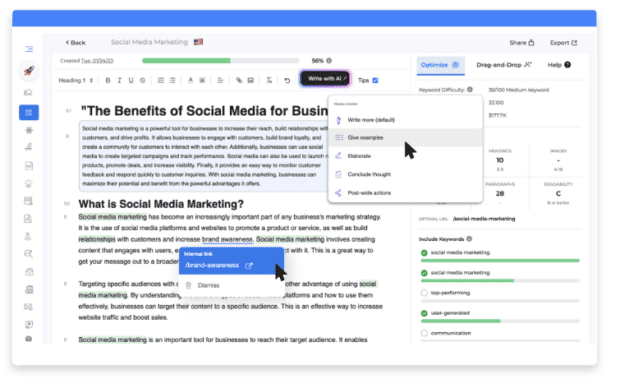
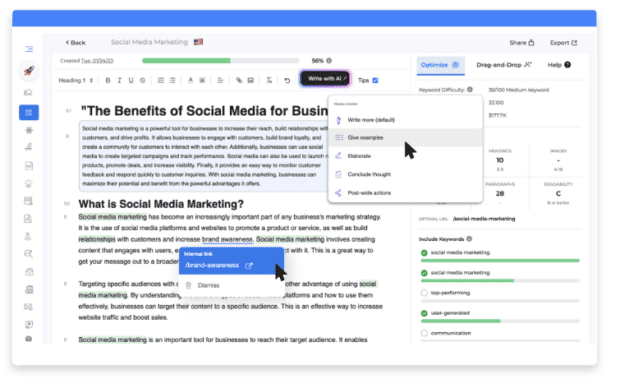
This tool excels in providing comprehensive backlinking strategies and creating outlines for blog post content. Bonus: Growthbar offers a Chrome extension for added convenience.
Price: From $36 a month
Who it’s best for: Content marketers, bloggers
19. Frase.io
Frase.io is a handy tool for creating SEO-optimized content efficiently.
Just enter a topic, and Frase automatically compares and extracts data from top sites using the same keyword. The AI-driven marketing tool then generates an SEO-friendly outline, allowing you to create content that’s more likely to rank in the SERPs.
Price: From $15 a month
Who it’s best for: Editors, content strategists, SEO experts
AI tools for advertising
20. Albert.ai
Albert positions itself as your “self-learning digital marketing ally,” with features that help it process and analyze audience info and tactical data at scale.
Albert is adept at optimizing and generating budgets. But it’s also designed to help with strategy and structure, so you can get more reach for less.
Price: Contact for a customized pricing plan
Who it’s best for: Digital marketers
21. Skai
If you’re in marketing in 2024, chances are you’re delivering to a bunch of different outlets and platforms. Skai is all about optimizing for an omnichannel strategy.
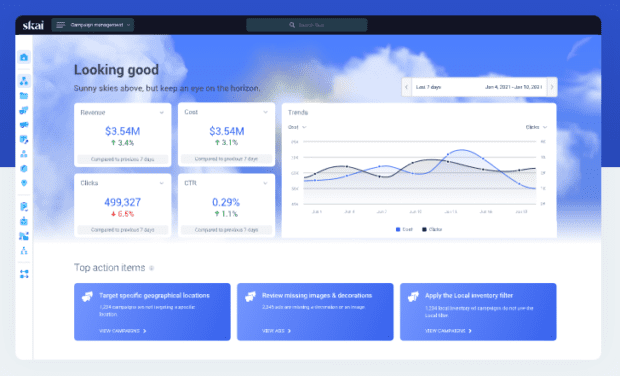
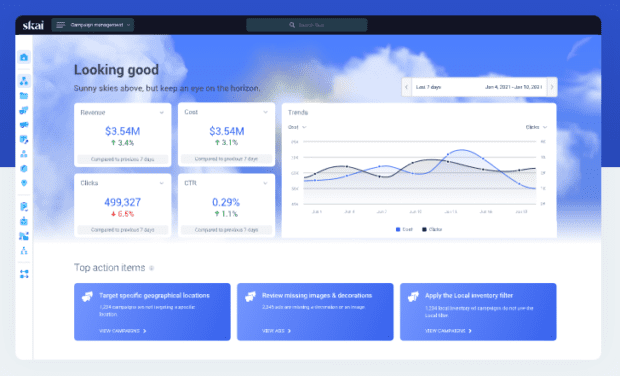
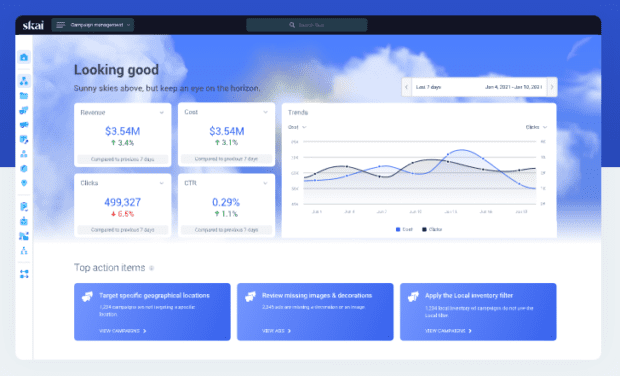
The tool uses AI-powered tech to collect and classify unstructured data points to gather insights about your unique market. Skai’s Creative Intelligence AI will even review your ad creative to offer feedback and tactics.
Price: Price on request
Who it’s best for: Omnichannel advertisers
22. Wordstream
Wordstream is another AI-based program dedicated to elevating your advertising game. It uses machine learning (ML) to optimize ad campaigns across a variety of social media networks.
Wordstream’s key features include ML-driven ad performances, cross-channel assessment of PPC ads, and comprehensive campaign analysis. It’s helpful whether you’re fine-tuning existing social media ads or crafting a new campaign from the ground up.
Price: Price on request
Who it’s best for: Small businesses, agencies
AI tools for market research
23. Brandwatch
Brandwatch is notable for its extensive reservoir of customer intelligence, specifically designed for analyzing data at scale.
Its AI capabilities generate valuable insights, statistics, and aggregated data. This streamlined approach allows users to spend less time deciphering data and more time implementing high-level takeaways.
One noteworthy feature: It can integrate with tools like ChatGPT, facilitating the production of natural language summaries for data sets.
Hootsuite users can take advantage of Hootsuite Insights powered by Brandwatch directly within the app, integrating Brandwatch’s search capabilities for a strategic advantage over competitors.
Price: Available with Hootsuite’s Enterprise accounts (request a demo now!)
Who it’s best for: Digital strategists, brand managers
24. Brand 24
Brand24’s AI social media monitoring tool allows brands to stay on top of real-time feedback, both positive and negative.
It conducts a comprehensive analysis of conversations spanning the web regarding the brand, products, and competitors so you can be as informed as humanly possible about the state of your reputation.
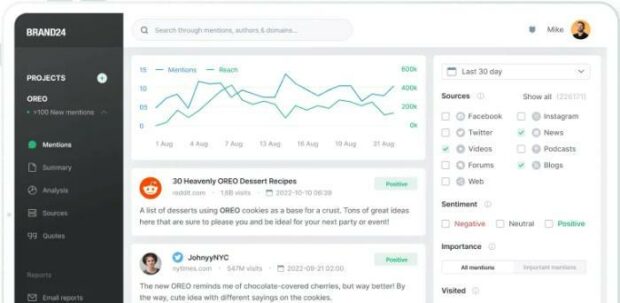
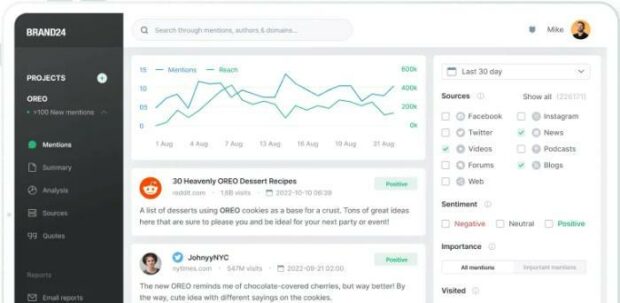
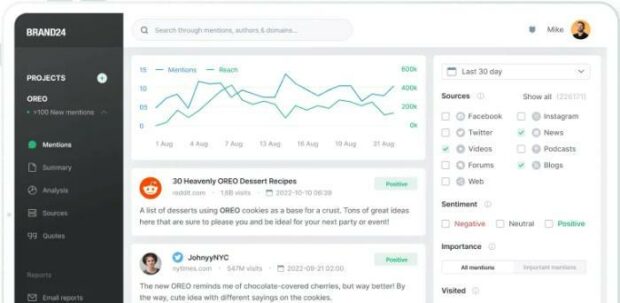
Beyond reputation management, Brand24 helps you assess ongoing marketing campaigns and resolve emerging issues before they escalate.
Price: From $99 a month
Who it’s best for: Brand managers, social media managers
25. Optimove
Optimove is a comprehensive customer data platform offering a unified view of customer behavior and insights.
Optimove features include campaign performance evaluation, hyper-segmentation, A/B testing, and multi-channel tracking. The tool can provide valuable insights, aiding in decisions about campaign optimization and managing customer exposure to marketing emails.
Price: Determined on the number of customers you have; get in touch for a quote
Who it’s best for: Customer relationship management professionals
Save time managing your social media presence with Hootsuite. From a single dashboard you can publish and schedule posts, find relevant conversions, engage the audience, measure results, and more. Try it free today.
Do it better with Hootsuite, the all-in-one social media tool. Stay on top of things, grow, and beat the competition.