Google Universal Analytics 360 Sunsetting Soon: Migration Tips & Top Alternative Inside via @sejournal, @PiwikPro
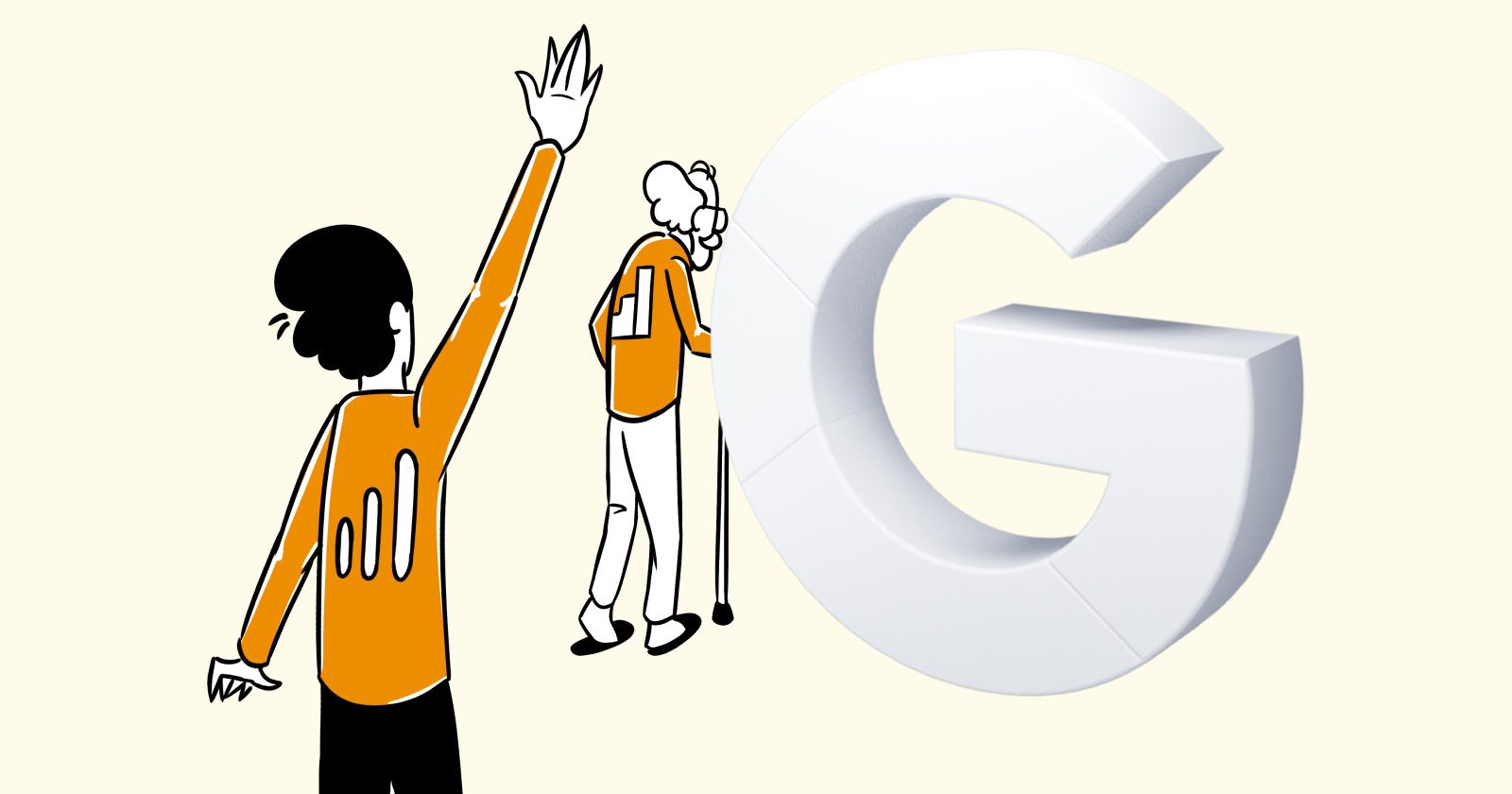
This post was sponsored by Piwik PRO. The opinions expressed in this article are the sponsor’s own.
This year, Google will finally phase out Universal Analytics 360, requiring paid users to switch to Google Analytics 360.
This is not something you can skip or postpone, and the clock is ticking.
The new analytics differ significantly from the previous version, and you can’t migrate data between them, so the transition can be challenging for organizations.
Since you’ll be starting from scratch, now is a good time to explore other options and determine if there are better solutions for your needs.
The three main areas to consider when deciding if you want to stay with Google or move to another platform are: the migration process, privacy and compliance, and ease of use.
When Is Google Universal Analytics 360 Sunsetting?
July 1, 2024 is when Google will phase out Universal Analytics 360.
What Should I Do Next?
Google encourages you to migrate to Google Analytics 360 as quickly as possible.
If you don’t, you could:
- Lose critical advertising capabilities.
- Lose the ability to export historical data.
- Face delays in setting up Google Analytics 360.
How To Migrate To Your Next Analytics Platform
Moving to a new platform is much more than just implementation; it is vital to plan your migration properly. Below are five steps to help you through the entire process.
Step 1. Evaluate Your Stack & Resources
Before you switch analytics tools, take the time to evaluate your entire stack, not just the tool you’re changing. Ensure that your stack is up-to-date and meets your current business needs. Migrating to a new analytics vendor almost always requires more people and more time than originally estimated. It’s a good occasion to remove redundant tools from your stack; it might also allow you to integrate with new ones that can help you run your analytics and collect data more comprehensively.
Step 2. Tidy Your Data
Over time, data collection may get messy, and you find yourself tracking data that isn’t relevant to your business. A migration gives you a chance to clean up your data taxonomy. Ensure that your new tool allows you to use the same categories of data as the previous one. Pay close attention to any data that needs to be collected automatically, like location data (country, region, city), and device details (device type, browser). Finally, make sure the SDKs you need are supported by your new tool.
Step 3. Implement A New Platform
This step involves setting up the tracking code that collects data about visitors to your website or app and making any necessary modifications. Remember to set up tags to gather more detailed data through events or connect third-party tools.
Speed Up The Transition: If you switch to Piwik PRO, you can use a migration tool to easily transfer your settings from Universal Analytics (GA3) and Google Tag Manager.
Step 4. Evaluate Tour New Data
Once you’re done implementing your new platform, you should run it parallel to your existing tool for a few months before finalizing the migration. During this time, you can audit your new data and correct any errors. In this manner, you can retain your historical data while simultaneously generating new data segments on the new platform.
Step 5. Provide Training For Your Team
All end users need training to comprehend the platform’s operations, retrieve necessary data, and generate reports. This step is frequently missed as it falls at the end of the project.
Upon finishing this step, you will be set to switch to your new platform fully. If you find the migration process challenging, consider getting help from outside sources. Some analytics vendors offer hands-on onboarding and user training, which accelerates product adoption.
Is Switching To Google Analytics 360 Worth The Hassle?
You might be thinking, “Migrating to the successor of UA 360 won’t be a walk in the park,” especially if you work for a large organization.
In addition to subscription and data migration costs, you may also need to train your staff or increase fees for external marketing agencies that will face new challenges.
While Analytics 360 has incredible use cases, there may be other tools that better suit your needs.
Switching to alternative solutions may be a good option for you.
How To Pick A Replacement For Universal Analytics 360
To decide whether to choose a new platform or stick with Google, consider a few important factors:
- Because GA 360 is a different software, your marketing and analytics departments will need to allocate extra resources to learn the new platform. You will also need the support of analysts, developers, and data architects to help you reconstruct reports based on the data architecture of the chosen platform. Choosing a solution with similar features and user experience to UA 360 can be a good option, because it saves resources, making onboarding faster and easier.
- You will also need to redesign your entire customer journey, because the data model in GA360 has changed from sessions to events. This process can be more challenging and costly than choosing a session-based platform or one that offers you freedom of choice.
- Another important consideration is the level of support offered by the vendor. This can greatly affect the quality of the migration and onboarding to a new platform. Although Google Analytics is currently the most popular tool for analyzing web traffic, the level of support it provides is limited. Other companies like Piwik PRO can offer more in this area, including personalized onboarding, product implementation, training, and dedicated customer support at every step.
Consideration 1: Think About Privacy & Compliance
Organizations around the world are increasingly concerned with data privacy and compliance. A 2023 Thomson survey found that 80% of business professionals acknowledge the importance of compliance as a crucial advisory function for their organizations. Gartner, on the other hand, predicts that, by 2025, 60% of large enterprises will use at least one privacy-enhancing computing (PEC) technique in analytics, business intelligence, and/or cloud computing.
This is due to a growing number of new regulations that place greater control over personal data at the forefront. The EU’s General Data Protection Regulation (GDPR) and the California Consumer Privacy Act (CCPA) are two of the most prominent examples. The landscape has been further complicated by events such as the Schrems II case, Brexit, and China’s Personal Data Protection Law. Data protection is also increasingly important in some sectors, such as healthcare, where regulations like HIPAA are mandatory.
If your company operates globally or has ambitions to do so, the first thing to consider is who has full ownership of the data, where the servers hosting the data are located, and who owns them. Google Analytics 360 only offers cloud deployment in an unknown location, which means that data might be transferred between data centers in the Americas, Europe, and Asia. This makes it difficult to know exactly where the data is stored and ownership is unclear. For now, the issue of data transfers between the US and the EU has been resolved by the EU-US Privacy Shield framework agreement, but the future stays unclear. Last year, NOYB, led by Max Schrems, announced that it would soon appeal this decision to the Court of Justice of the European Union (CJEU).
To meet privacy and compliance requirements in different countries and industries, choose a platform that allows you to customize your hosting plan and set specific parameters for data collection and analysis. Platforms like Piwik PRO Analytics Suite enable you to store your data on servers in Europe, the US, and Asia, based on your preferences. This translates into flexibility and security of your data.
Consideration 2: Ease Of Use & Integration
This may sound counterintuitive, but the new GA 360 might be too complex for many. While it offers numerous advanced functions for data analysts, it lacks features specifically designed for marketers. As a result, marketers may need help in configuring the system to efficiently use the data.
On the other hand, in GA 360, the data model shifts from session-based to event-based. This is especially important if your teams depend on UA 360 behavioral reporting, benchmarking, and e-commerce flow reports, as these features are unavailable in the new release. You also need to revise all the reports for all the stakeholders.
Conversely, Piwik PRO strongly emphasizes simplicity and enables marketers to quickly access the necessary data. Additionally, the data model combines both session-based and event-based structures. This approach ensures that you can start working with the data faster and deliver the reports that stakeholders are used to. Another big advantage of Piwik PRO is its model for working with raw data, which is a valuable source of knowledge about users and provides richer reporting in more contexts. Google Analytics does not provide raw data exports, so you have to use various services and tools to accomplish this. To be fair, however, exporting large raw data packets with Piwik PRO software may take longer than with Google solutions.
The new GA 360 is most effective when used mainly with products from the Google ecosystem. When considering data activation, Google Ads is the most suitable option. When it comes to Piwik PRO, you still have this option, but integrating with other solutions is much easier. The platform offers four modules: Analytics, Tag Manager, Consent Manager and Customer Data Platform (CDP). The CDP module, available in the paid plan, lets you create detailed customer profiles and categorize your data into various audience segments. You can activate them to provide a personalized experience and run effective campaigns across multiple channels.
The landscape of modern analytics is constantly changing. On the one hand, there are ongoing discussions about privacy and compliance regulations, while on the other, companies are trying out various methods to collect and analyze data. In the end, your choice of analytics platform will impact the performance of your marketing and sales efforts. So take the time to explore, and you may find other solutions that better suit your organization’s needs.
Piwik PRO is a solid choice to explore for your next primary analytics solution. Book a personalized demo of the Enterprise version and see the benefits of introducing Piwik PRO Analytics Suite in your organization.
Image Credits
Featured Image: Image by Piwik PRO Used with permission.